Our Research
Research
Wenhao Chai
My current research focus on developing embodied intelligence inspired by cognitive science principles to interact with the physical world, building upon video understanding as a core perceptual mechanism. I propose a long-short term memory framework modeled after the human memory system, enabling pre-trained video LMMs to comprehend multi-hour video content without additional fine-tuning. To enhance efficiency, I introduce token merging, significantly reducing visual tokens with minimal performance degradation. I also demonstrate step-by-step agent system development in Minecraft, showcasing cognitive-inspired agent capabilities in virtual environments.
I have just begun shaping my research narrative around embodied intelligence and cognitive science. With "pretraining as we know it will end", I believe the future of artificial intelligence lies in aligning with human intelligence—and ultimately surpassing it.
The following showcases my complete past research experience and fields with timeline. The template is from here.
How to efficiently build and evaluate large multi-modal models?
How to involve large multi-modal models in embodied agent system?
-
Video Understanding
- Long Video with Long-Short Term Memory [MovieChat]
- Long Video with Language Guidance Memory [MovieChat+]
- Video Detailed Captioning [AuroraCap]
-
Embodied Agent
- Build in Minecraft [STEVE-1]
- Multi-Agent [STEVE-1.5]
- + Knowledge Distillation [STEVE-2]
How to generate high-quality images, videos and 3D worlds?
How to control and evaluate the generated content?
-
Video
-
Image
-
3D World
How to estimate human pose and motion from images and videos?
How to generate realistic and controllable human motion?
-
3D Human Pose Estimation
-
Motion Representation
-
Tracking
Featured
Videos
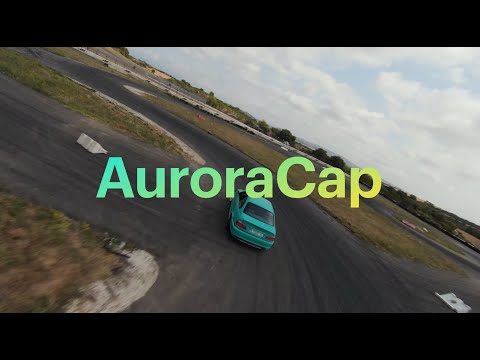
AuroraCap [Project Page]
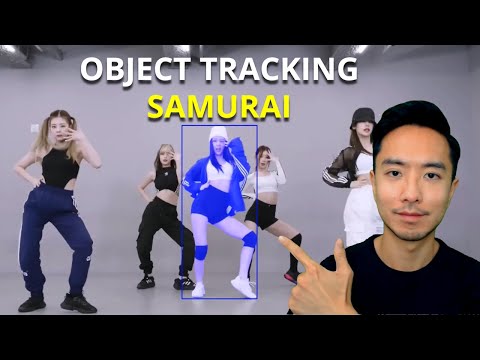
SAMURAI [Project Page]
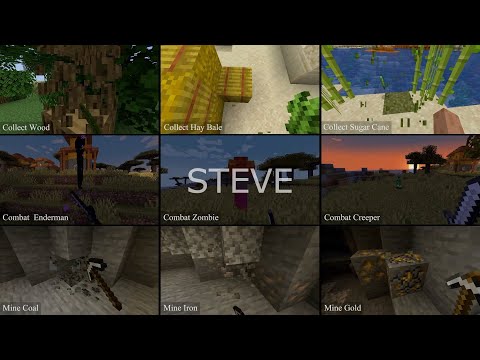
STEVE [Project Page]
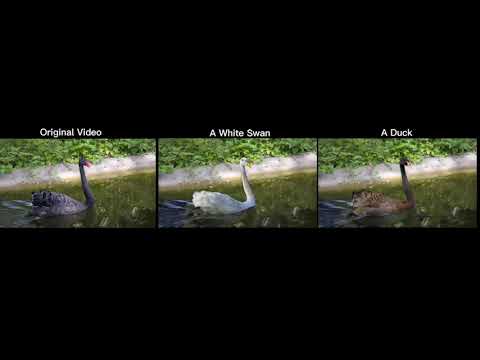
StableVideo [Hugging Face Demo]
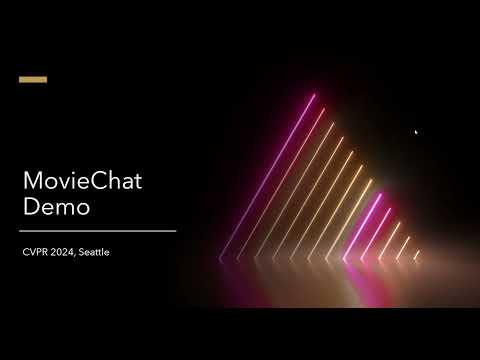
MovieChat [Project Page]
Organized
Workshops | Tutorials | Talks
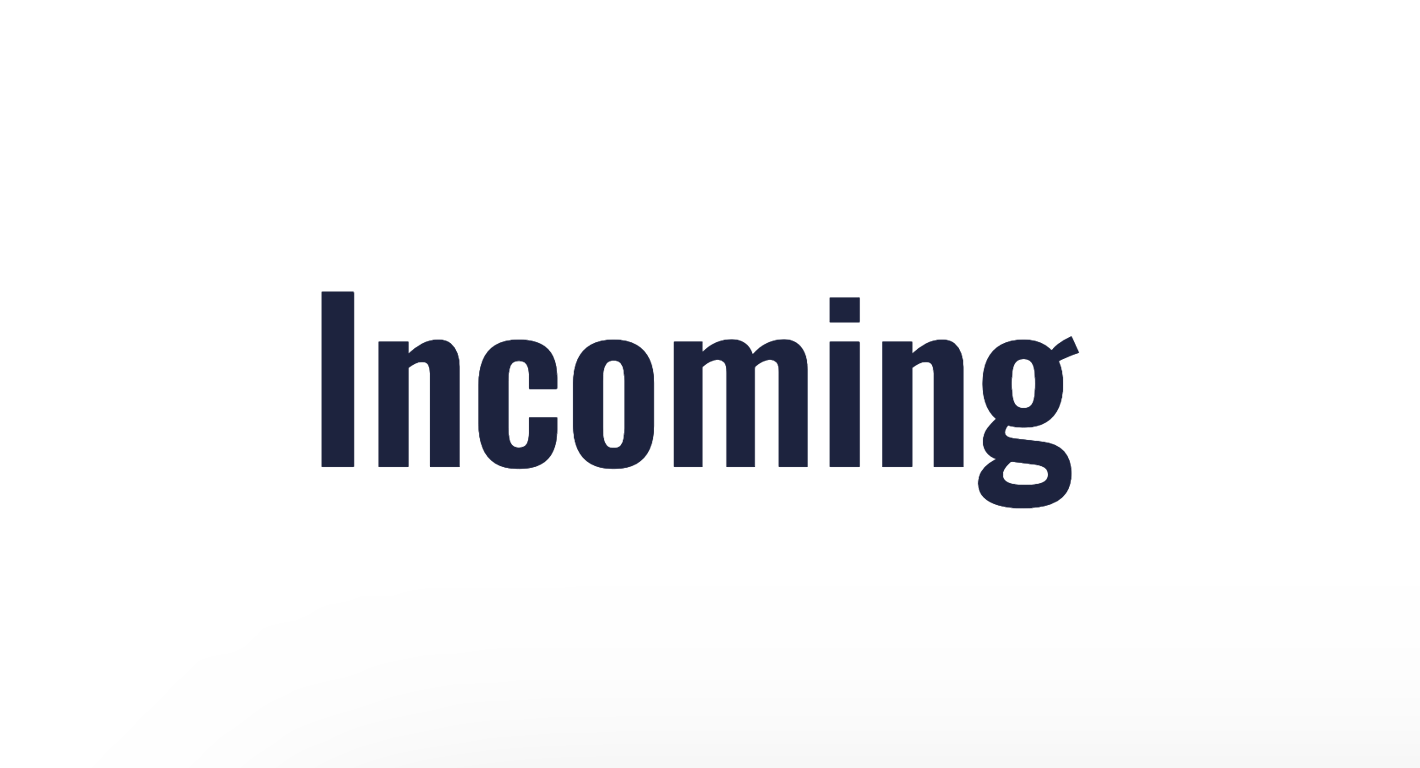
5th International Workshop on Long-form Video Understanding: Towards Multimodal AI Assistant and Copilot
CVPR 2025, Nashville, TN
Workshop Organizer
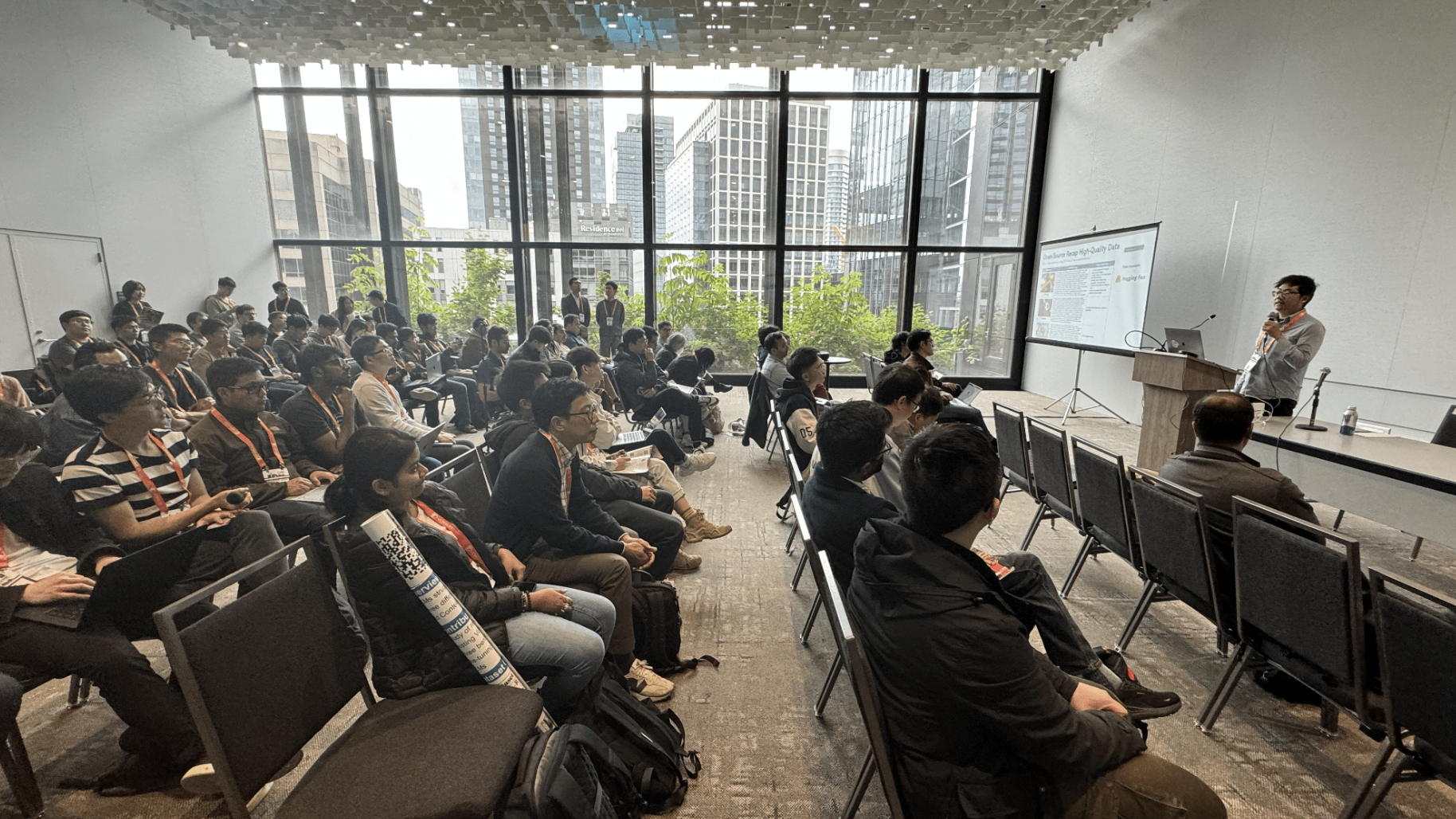
4th International Workshop on Long-form Video Understanding: Towards Multimodal AI Assistant and Copilot
CVPR 2024, Seattle, WA
Workshop Organizer (Track 1)
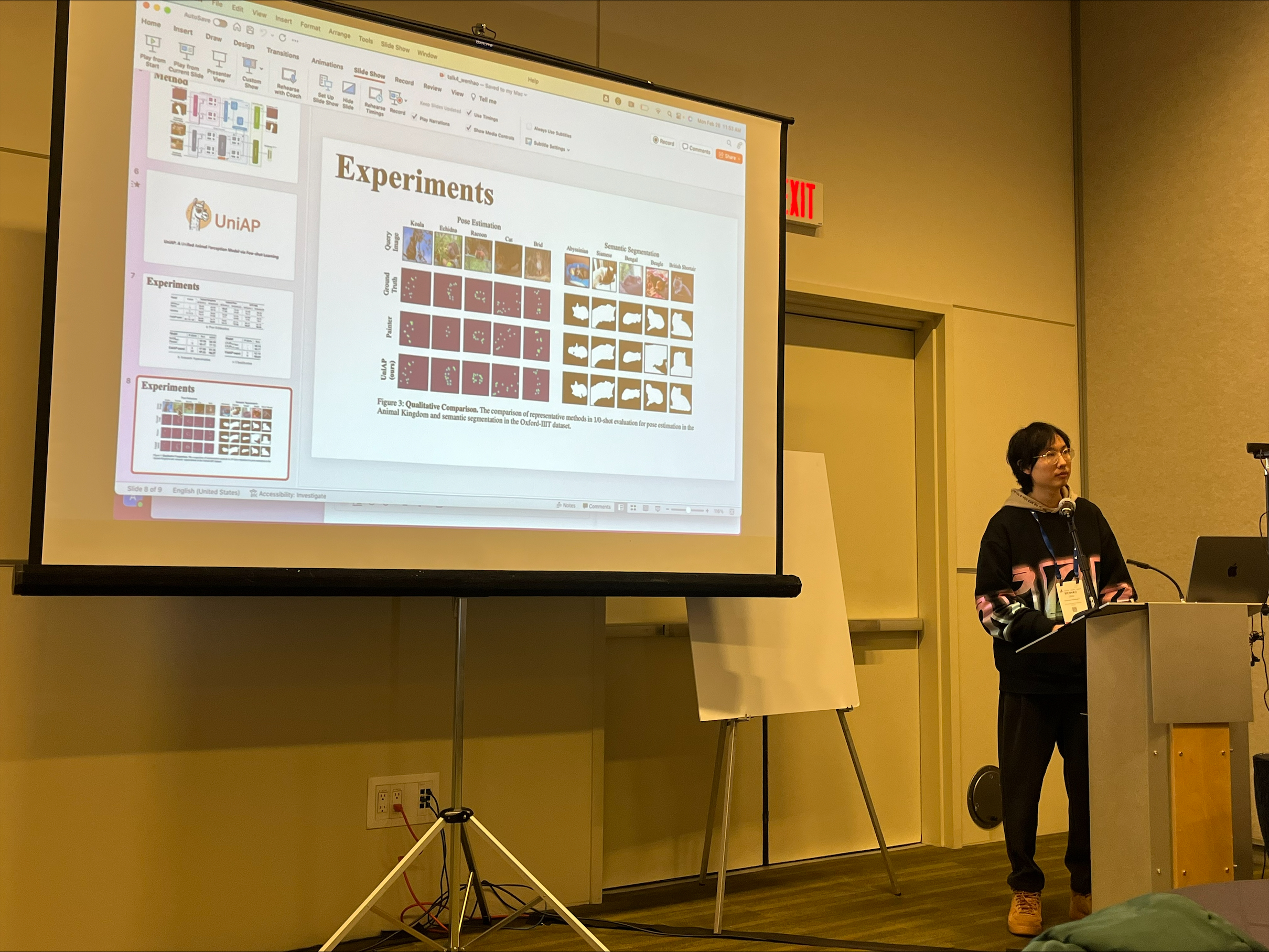
First Workshop on Imageomics: Discovering Biological Knowledge from Images using AI
AAAI 2024, Vancouver, Canada
Invited Talk
Featured
Posters
† Attend in person.
Ego3DT: Tracking Every 3D Object in Ego-centric Videos
ACM MM 2024, Melbourne, Australia
† MovieChat: From Dense Token to Sparse Memory for Long Video Understanding
CVPR 2024, Seattle, WA
† STEVE Series: Step-by-Step Construction of Agent Systems in Minecraft
CVPR 2024 workshop, Seattle, WA
† UniAP: Towards Universal Animal Perception in Vision via Few-shot Learning
AAAI 2024, Vancouver, Canada
† StableVideo: Text-driven Consistency-aware Diffusion Video Editing
ICCV 2023, Paris, France
See and Think: Embodied Agent in Virtual Environment
ECCV 2024, Milano, Italy
† Learning Diffusion Texture Priors for Image Restoration
CVPR 2024, Seattle, WA
Hierarchical Auto-Organizing System for Open-Ended Multi-Agent Navigation
ICLR 2024 workshop, Vienna, Austria
† Back to Optimization: Diffusion-based Zero-Shot 3D Human Pose Estimation
WACV 2024, Waikoloa, Hawaii
† Global Adaptation meets Local Generalization: Unsupervised Domain Adaptation for 3D Human Pose Estimation
ICCV 2023, Paris, France